Assista agora Este tutorial tem um curso em vídeo relacionado criado pela equipe Real Python. Assista junto com o tutorial escrito para aprofundar sua compreensão:Ler e escrever arquivos com Pandas
Pandas é um pacote Python poderoso e flexível que permite trabalhar com dados rotulados e de séries temporais. Ele também fornece métodos estatísticos, permite plotagem e muito mais. Um recurso crucial do Pandas é sua capacidade de escrever e ler Excel, CSV e muitos outros tipos de arquivos. Funções como os Pandas
read_csv()
permitem que você trabalhe com arquivos de forma eficaz. Você pode usá-los para salvar os dados e rótulos dos objetos Pandas em um arquivo e carregá-los posteriormente como Pandas Series
ou DataFrame
instâncias. Neste tutorial, você aprenderá:
- O que as ferramentas Pandas IO API é
- Como ler e gravar dados de e para arquivos
- Como trabalhar com vários formatos de arquivo
- Como trabalhar com big data eficiente
Vamos começar a ler e escrever arquivos!
Bônus grátis: 5 Pensamentos sobre o domínio do Python, um curso gratuito para desenvolvedores de Python que mostra o roteiro e a mentalidade de que você precisa para levar suas habilidades de Python para o próximo nível.
Instalando Pandas
O código neste tutorial é executado com CPython 3.7.4 e Pandas 0.25.1. Seria benéfico garantir que você tenha as versões mais recentes do Python e do Pandas em sua máquina. Você pode querer criar um novo ambiente virtual e instalar as dependências para este tutorial.
Primeiro, você precisará da biblioteca Pandas. Você talvez já tenha instalado isso. Se você não fizer isso, você pode instalá-lo com pip:
$ pip install pandas
Quando o processo de instalação estiver concluído, você deverá ter o Pandas instalado e pronto.
Anaconda é uma excelente distribuição Python que vem com Python, muitos pacotes úteis como Pandas e um gerenciador de pacotes e ambiente chamado Conda. Para saber mais sobre o Anaconda, confira Configurando o Python para Machine Learning no Windows.
Se você não tiver Pandas em seu ambiente virtual, poderá instalá-lo com o Conda:
$ conda install pandas
O Conda é poderoso, pois gerencia as dependências e suas versões. Para saber mais sobre como trabalhar com o Conda, você pode conferir a documentação oficial.
Preparando dados
Neste tutorial, você usará os dados relacionados a 20 países. Aqui está uma visão geral dos dados e das fontes com as quais você trabalhará:
-
País é indicado pelo nome do país. Cada país está na lista dos 10 primeiros em população, área ou produto interno bruto (PIB). Os rótulos de linha para o conjunto de dados são os códigos de país de três letras definidos na ISO 3166-1. O rótulo da coluna para o conjunto de dados éCOUNTRY
.
-
População é expresso em milhões. Os dados vêm de uma lista de países e dependências por população na Wikipedia. O rótulo da coluna para o conjunto de dados éPOP
.
-
Área é expresso em milhares de quilômetros quadrados. Os dados vêm de uma lista de países e dependências por área na Wikipedia. O rótulo da coluna para o conjunto de dados éAREA
.
-
Produto interno bruto é expresso em milhões de dólares americanos, de acordo com os dados das Nações Unidas para 2017. Você pode encontrar esses dados na lista de países por PIB nominal na Wikipedia. O rótulo da coluna para o conjunto de dados éGDP
.
-
Continente é África, Ásia, Oceania, Europa, América do Norte ou América do Sul. Você também pode encontrar essas informações na Wikipedia. O rótulo da coluna para o conjunto de dados éCONT
.
-
Dia da Independência é uma data que comemora a independência de uma nação. Os dados vêm da lista de dias da independência nacional na Wikipedia. As datas são mostradas no formato ISO 8601. Os primeiros quatro dígitos representam o ano, os próximos dois números são o mês e os dois últimos são para o dia do mês. O rótulo da coluna para o conjunto de dados éIND_DAY
.
Esta é a aparência dos dados como uma tabela:
PAÍS | POP | ÁREA | PIB | CONT | IND_DAY | |
---|---|---|---|---|---|---|
CHN | China | 1398,72 | 9596,96 | 12234,78 | Ásia | |
IND | Índia | 1351,16 | 3287,26 | 2575,67 | Ásia | 15-08-1947 |
EUA | EUA | 329,74 | 9833,52 | 19485.39 | N.América | 1776-07-04 |
IDN | Indonésia | 268,07 | 1910,93 | 1015,54 | Ásia | 17-08-1945 |
SUTIÃ | Brasil | 210,32 | 8515,77 | 2055,51 | S.América | 1822-09-07 |
PAK | Paquistão | 205,71 | 881,91 | 302.14 | Ásia | 14-08-1947 |
NGA | Nigéria | 200,96 | 923,77 | 375,77 | África | 1960-10-01 |
BGD | Bangladesh | 167,09 | 147,57 | 245,63 | Ásia | 1971-03-26 |
RUS | Rússia | 146,79 | 17098,25 | 1530,75 | 12/06/1992 | |
MEX | México | 126,58 | 1964,38 | 1158,23 | N.América | 1810-09-16 |
JPN | Japão | 126,22 | 377,97 | 4872,42 | Ásia | |
DEU | Alemanha | 83,02 | 357,11 | 3693,20 | Europa | |
FRA | França | 67,02 | 640,68 | 2582,49 | Europa | 1789-07-14 |
GBR | Reino Unido | 66,44 | 242,50 | 2631,23 | Europa | |
ITA | Itália | 60,36 | 301,34 | 1943,84 | Europa | |
ARG | Argentina | 44,94 | 2780,40 | 637,49 | S.América | 1816-07-09 |
DZA | Argélia | 43,38 | 2381,74 | 167,56 | África | 1962-07-05 |
PODE | Canadá | 37,59 | 9984,67 | 1647.12 | N.América | 1867-07-01 |
AUS | Austrália | 25,47 | 7692.02 | 1408,68 | Oceânia | |
KAZ | Cazaquistão | 18,53 | 2724,90 | 159,41 | Ásia | 16-12-1991 |
Você pode notar que alguns dos dados estão faltando. Por exemplo, o continente da Rússia não é especificado porque se espalha pela Europa e Ásia. Há também vários dias de independência ausentes porque a fonte de dados os omite.
Você pode organizar esses dados em Python usando um dicionário aninhado:
data = {
'CHN': {'COUNTRY': 'China', 'POP': 1_398.72, 'AREA': 9_596.96,
'GDP': 12_234.78, 'CONT': 'Asia'},
'IND': {'COUNTRY': 'India', 'POP': 1_351.16, 'AREA': 3_287.26,
'GDP': 2_575.67, 'CONT': 'Asia', 'IND_DAY': '1947-08-15'},
'USA': {'COUNTRY': 'US', 'POP': 329.74, 'AREA': 9_833.52,
'GDP': 19_485.39, 'CONT': 'N.America',
'IND_DAY': '1776-07-04'},
'IDN': {'COUNTRY': 'Indonesia', 'POP': 268.07, 'AREA': 1_910.93,
'GDP': 1_015.54, 'CONT': 'Asia', 'IND_DAY': '1945-08-17'},
'BRA': {'COUNTRY': 'Brazil', 'POP': 210.32, 'AREA': 8_515.77,
'GDP': 2_055.51, 'CONT': 'S.America', 'IND_DAY': '1822-09-07'},
'PAK': {'COUNTRY': 'Pakistan', 'POP': 205.71, 'AREA': 881.91,
'GDP': 302.14, 'CONT': 'Asia', 'IND_DAY': '1947-08-14'},
'NGA': {'COUNTRY': 'Nigeria', 'POP': 200.96, 'AREA': 923.77,
'GDP': 375.77, 'CONT': 'Africa', 'IND_DAY': '1960-10-01'},
'BGD': {'COUNTRY': 'Bangladesh', 'POP': 167.09, 'AREA': 147.57,
'GDP': 245.63, 'CONT': 'Asia', 'IND_DAY': '1971-03-26'},
'RUS': {'COUNTRY': 'Russia', 'POP': 146.79, 'AREA': 17_098.25,
'GDP': 1_530.75, 'IND_DAY': '1992-06-12'},
'MEX': {'COUNTRY': 'Mexico', 'POP': 126.58, 'AREA': 1_964.38,
'GDP': 1_158.23, 'CONT': 'N.America', 'IND_DAY': '1810-09-16'},
'JPN': {'COUNTRY': 'Japan', 'POP': 126.22, 'AREA': 377.97,
'GDP': 4_872.42, 'CONT': 'Asia'},
'DEU': {'COUNTRY': 'Germany', 'POP': 83.02, 'AREA': 357.11,
'GDP': 3_693.20, 'CONT': 'Europe'},
'FRA': {'COUNTRY': 'France', 'POP': 67.02, 'AREA': 640.68,
'GDP': 2_582.49, 'CONT': 'Europe', 'IND_DAY': '1789-07-14'},
'GBR': {'COUNTRY': 'UK', 'POP': 66.44, 'AREA': 242.50,
'GDP': 2_631.23, 'CONT': 'Europe'},
'ITA': {'COUNTRY': 'Italy', 'POP': 60.36, 'AREA': 301.34,
'GDP': 1_943.84, 'CONT': 'Europe'},
'ARG': {'COUNTRY': 'Argentina', 'POP': 44.94, 'AREA': 2_780.40,
'GDP': 637.49, 'CONT': 'S.America', 'IND_DAY': '1816-07-09'},
'DZA': {'COUNTRY': 'Algeria', 'POP': 43.38, 'AREA': 2_381.74,
'GDP': 167.56, 'CONT': 'Africa', 'IND_DAY': '1962-07-05'},
'CAN': {'COUNTRY': 'Canada', 'POP': 37.59, 'AREA': 9_984.67,
'GDP': 1_647.12, 'CONT': 'N.America', 'IND_DAY': '1867-07-01'},
'AUS': {'COUNTRY': 'Australia', 'POP': 25.47, 'AREA': 7_692.02,
'GDP': 1_408.68, 'CONT': 'Oceania'},
'KAZ': {'COUNTRY': 'Kazakhstan', 'POP': 18.53, 'AREA': 2_724.90,
'GDP': 159.41, 'CONT': 'Asia', 'IND_DAY': '1991-12-16'}
}
columns = ('COUNTRY', 'POP', 'AREA', 'GDP', 'CONT', 'IND_DAY')
Cada linha da tabela é escrita como um dicionário interno cujas chaves são os nomes das colunas e os valores são os dados correspondentes. Esses dicionários são então coletados como os valores nos
data
externos dicionário. As chaves correspondentes para data
são os códigos de país de três letras. Você pode usar estes
data
para criar uma instância de um Pandas DataFrame
. Primeiro, você precisa importar Pandas:>>>
>>> import pandas as pd
Agora que você importou o Pandas, você pode usar o
DataFrame
construtor e data
para criar um DataFrame
objeto. data
está organizado de forma que os códigos dos países correspondam às colunas. Você pode reverter as linhas e colunas de um DataFrame
com a propriedade .T
:>>>
>>> df = pd.DataFrame(data=data).T
>>> df
COUNTRY POP AREA GDP CONT IND_DAY
CHN China 1398.72 9596.96 12234.8 Asia NaN
IND India 1351.16 3287.26 2575.67 Asia 1947-08-15
USA US 329.74 9833.52 19485.4 N.America 1776-07-04
IDN Indonesia 268.07 1910.93 1015.54 Asia 1945-08-17
BRA Brazil 210.32 8515.77 2055.51 S.America 1822-09-07
PAK Pakistan 205.71 881.91 302.14 Asia 1947-08-14
NGA Nigeria 200.96 923.77 375.77 Africa 1960-10-01
BGD Bangladesh 167.09 147.57 245.63 Asia 1971-03-26
RUS Russia 146.79 17098.2 1530.75 NaN 1992-06-12
MEX Mexico 126.58 1964.38 1158.23 N.America 1810-09-16
JPN Japan 126.22 377.97 4872.42 Asia NaN
DEU Germany 83.02 357.11 3693.2 Europe NaN
FRA France 67.02 640.68 2582.49 Europe 1789-07-14
GBR UK 66.44 242.5 2631.23 Europe NaN
ITA Italy 60.36 301.34 1943.84 Europe NaN
ARG Argentina 44.94 2780.4 637.49 S.America 1816-07-09
DZA Algeria 43.38 2381.74 167.56 Africa 1962-07-05
CAN Canada 37.59 9984.67 1647.12 N.America 1867-07-01
AUS Australia 25.47 7692.02 1408.68 Oceania NaN
KAZ Kazakhstan 18.53 2724.9 159.41 Asia 1991-12-16
Agora você tem seu
DataFrame
objeto preenchido com os dados sobre cada país. Observação: Você pode usar
.transpose()
em vez de .T
para reverter as linhas e colunas do seu conjunto de dados. Se você usar .transpose()
, então você pode definir o parâmetro opcional copy
para especificar se você deseja copiar os dados subjacentes. O comportamento padrão é False
. Versões do Python anteriores à 3.6 não garantiam a ordem das chaves nos dicionários. Para garantir que a ordem das colunas seja mantida para versões mais antigas do Python e Pandas, você pode especificar
index=columns
:>>>
>>> df = pd.DataFrame(data=data, index=columns).T
Agora que você preparou seus dados, está pronto para começar a trabalhar com arquivos!
Usando os Pandas read_csv()
e .to_csv()
Funções
Um arquivo de valores separados por vírgula (CSV) é um arquivo de texto simples com um
.csv
extensão que contém dados tabulares. Este é um dos formatos de arquivo mais populares para armazenar grandes quantidades de dados. Cada linha do arquivo CSV representa uma única linha da tabela. Os valores na mesma linha são separados por vírgulas por padrão, mas você pode alterar o separador para um ponto e vírgula, tabulação, espaço ou algum outro caractere. Escrever um arquivo CSV
Você pode salvar seus Pandas
DataFrame
como um arquivo CSV com .to_csv()
:>>>
>>> df.to_csv('data.csv')
É isso! Você criou o arquivo
data.csv
em seu diretório de trabalho atual. Você pode expandir o bloco de código abaixo para ver como seu arquivo CSV deve ficar:,COUNTRY,POP,AREA,GDP,CONT,IND_DAY
CHN,China,1398.72,9596.96,12234.78,Asia,
IND,India,1351.16,3287.26,2575.67,Asia,1947-08-15
USA,US,329.74,9833.52,19485.39,N.America,1776-07-04
IDN,Indonesia,268.07,1910.93,1015.54,Asia,1945-08-17
BRA,Brazil,210.32,8515.77,2055.51,S.America,1822-09-07
PAK,Pakistan,205.71,881.91,302.14,Asia,1947-08-14
NGA,Nigeria,200.96,923.77,375.77,Africa,1960-10-01
BGD,Bangladesh,167.09,147.57,245.63,Asia,1971-03-26
RUS,Russia,146.79,17098.25,1530.75,,1992-06-12
MEX,Mexico,126.58,1964.38,1158.23,N.America,1810-09-16
JPN,Japan,126.22,377.97,4872.42,Asia,
DEU,Germany,83.02,357.11,3693.2,Europe,
FRA,France,67.02,640.68,2582.49,Europe,1789-07-14
GBR,UK,66.44,242.5,2631.23,Europe,
ITA,Italy,60.36,301.34,1943.84,Europe,
ARG,Argentina,44.94,2780.4,637.49,S.America,1816-07-09
DZA,Algeria,43.38,2381.74,167.56,Africa,1962-07-05
CAN,Canada,37.59,9984.67,1647.12,N.America,1867-07-01
AUS,Australia,25.47,7692.02,1408.68,Oceania,
KAZ,Kazakhstan,18.53,2724.9,159.41,Asia,1991-12-16
Este arquivo de texto contém os dados separados por vírgulas . A primeira coluna contém os rótulos das linhas. Em alguns casos, você os achará irrelevantes. Se você não quiser mantê-los, você pode passar o argumento
index=False
para .to_csv()
. Ler um arquivo CSV
Depois que seus dados forem salvos em um arquivo CSV, você provavelmente desejará carregá-los e usá-los de tempos em tempos. Você pode fazer isso com os Pandas
read_csv()
função:>>>
>>> df = pd.read_csv('data.csv', index_col=0)
>>> df
COUNTRY POP AREA GDP CONT IND_DAY
CHN China 1398.72 9596.96 12234.78 Asia NaN
IND India 1351.16 3287.26 2575.67 Asia 1947-08-15
USA US 329.74 9833.52 19485.39 N.America 1776-07-04
IDN Indonesia 268.07 1910.93 1015.54 Asia 1945-08-17
BRA Brazil 210.32 8515.77 2055.51 S.America 1822-09-07
PAK Pakistan 205.71 881.91 302.14 Asia 1947-08-14
NGA Nigeria 200.96 923.77 375.77 Africa 1960-10-01
BGD Bangladesh 167.09 147.57 245.63 Asia 1971-03-26
RUS Russia 146.79 17098.25 1530.75 NaN 1992-06-12
MEX Mexico 126.58 1964.38 1158.23 N.America 1810-09-16
JPN Japan 126.22 377.97 4872.42 Asia NaN
DEU Germany 83.02 357.11 3693.20 Europe NaN
FRA France 67.02 640.68 2582.49 Europe 1789-07-14
GBR UK 66.44 242.50 2631.23 Europe NaN
ITA Italy 60.36 301.34 1943.84 Europe NaN
ARG Argentina 44.94 2780.40 637.49 S.America 1816-07-09
DZA Algeria 43.38 2381.74 167.56 Africa 1962-07-05
CAN Canada 37.59 9984.67 1647.12 N.America 1867-07-01
AUS Australia 25.47 7692.02 1408.68 Oceania NaN
KAZ Kazakhstan 18.53 2724.90 159.41 Asia 1991-12-16
Neste caso, os Pandas
read_csv()
função retorna um novo DataFrame
com os dados e rótulos do arquivo data.csv
, que você especificou com o primeiro argumento. Essa string pode ser qualquer caminho válido, incluindo URLs. O parâmetro
index_col
especifica a coluna do arquivo CSV que contém os rótulos de linha. Você atribui um índice de coluna baseado em zero a esse parâmetro. Você deve determinar o valor de index_col
quando o arquivo CSV contém os rótulos de linha para evitar carregá-los como dados. Você aprenderá mais sobre como usar Pandas com arquivos CSV posteriormente neste tutorial. Você também pode conferir Lendo e gravando arquivos CSV em Python para ver como lidar com arquivos CSV com a biblioteca Python integrada csv.
Usando Pandas para escrever e ler arquivos do Excel
O Microsoft Excel é provavelmente o software de planilha mais utilizado. Enquanto as versões mais antigas usavam o binário
.xls
arquivos, o Excel 2007 introduziu o novo .xlsx
baseado em XML Arquivo. Você pode ler e gravar arquivos do Excel no Pandas, semelhante aos arquivos CSV. No entanto, você precisará instalar os seguintes pacotes Python primeiro:- xlwt para gravar em
.xls
arquivos - openpyxl ou XlsxWriter para gravar em
.xlsx
arquivos - xlrd para ler arquivos do Excel
Você pode instalá-los usando pip com um único comando:
$ pip install xlwt openpyxl xlsxwriter xlrd
Você também pode usar o Conda:
$ conda install xlwt openpyxl xlsxwriter xlrd
Observe que você não precisa instalar todos esses pacotes. Por exemplo, você não precisa de openpyxl e XlsxWriter. Se você for trabalhar apenas com
.xls
arquivos, então você não precisa de nenhum deles! No entanto, se você pretende trabalhar apenas com .xlsx
arquivos, então você precisará de pelo menos um deles, mas não de xlwt
. Reserve algum tempo para decidir quais pacotes são adequados para o seu projeto. Escrever um arquivo Excel
Depois de instalar esses pacotes, você pode salvar seu
DataFrame
em um arquivo Excel com .to_excel()
:>>>
>>> df.to_excel('data.xlsx')
O argumento
'data.xlsx'
representa o arquivo de destino e, opcionalmente, seu caminho. A instrução acima deve criar o arquivo data.xlsx
em seu diretório de trabalho atual. Esse arquivo deve ficar assim: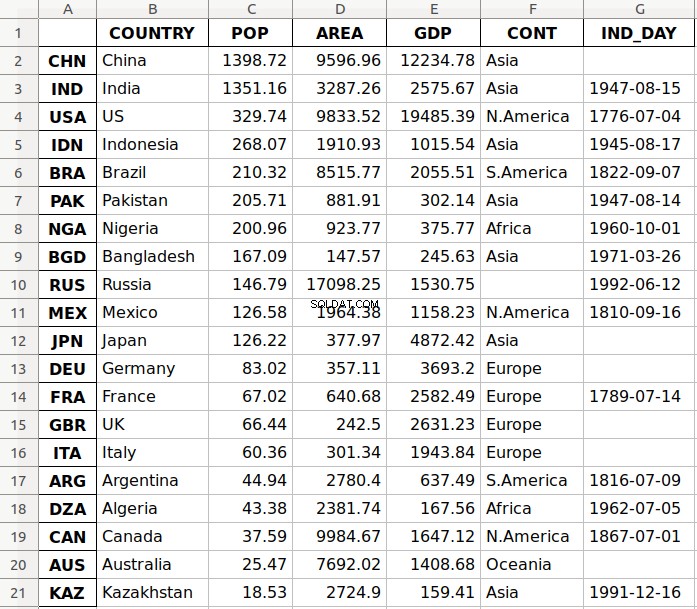
A primeira coluna do arquivo contém os rótulos das linhas, enquanto as outras colunas armazenam os dados.
Ler um arquivo do Excel
Você pode carregar dados de arquivos do Excel com
read_excel()
:>>>
>>> df = pd.read_excel('data.xlsx', index_col=0)
>>> df
COUNTRY POP AREA GDP CONT IND_DAY
CHN China 1398.72 9596.96 12234.78 Asia NaN
IND India 1351.16 3287.26 2575.67 Asia 1947-08-15
USA US 329.74 9833.52 19485.39 N.America 1776-07-04
IDN Indonesia 268.07 1910.93 1015.54 Asia 1945-08-17
BRA Brazil 210.32 8515.77 2055.51 S.America 1822-09-07
PAK Pakistan 205.71 881.91 302.14 Asia 1947-08-14
NGA Nigeria 200.96 923.77 375.77 Africa 1960-10-01
BGD Bangladesh 167.09 147.57 245.63 Asia 1971-03-26
RUS Russia 146.79 17098.25 1530.75 NaN 1992-06-12
MEX Mexico 126.58 1964.38 1158.23 N.America 1810-09-16
JPN Japan 126.22 377.97 4872.42 Asia NaN
DEU Germany 83.02 357.11 3693.20 Europe NaN
FRA France 67.02 640.68 2582.49 Europe 1789-07-14
GBR UK 66.44 242.50 2631.23 Europe NaN
ITA Italy 60.36 301.34 1943.84 Europe NaN
ARG Argentina 44.94 2780.40 637.49 S.America 1816-07-09
DZA Algeria 43.38 2381.74 167.56 Africa 1962-07-05
CAN Canada 37.59 9984.67 1647.12 N.America 1867-07-01
AUS Australia 25.47 7692.02 1408.68 Oceania NaN
KAZ Kazakhstan 18.53 2724.90 159.41 Asia 1991-12-16
read_excel()
retorna um novo DataFrame
que contém os valores de data.xlsx
. Você também pode usar read_excel()
com planilhas OpenDocument ou .ods
arquivos. Você aprenderá mais sobre como trabalhar com arquivos do Excel posteriormente neste tutorial. Você também pode conferir Usando Pandas para Ler Arquivos Grandes do Excel em Python.
Compreendendo a API Pandas IO
Ferramentas Pandas IO é a API que permite salvar o conteúdo de
Series
e DataFrame
objetos para a área de transferência, objetos ou arquivos de vários tipos. Também permite carregar dados da área de transferência, objetos ou arquivos. Escrever arquivos
Series
e DataFrame
os objetos têm métodos que permitem gravar dados e rótulos na área de transferência ou nos arquivos. Eles são nomeados com o padrão .to_<file-type>()
, onde <file-type>
é o tipo do arquivo de destino. Você aprendeu sobre
.to_csv()
e .to_excel()
, mas existem outros, incluindo:.to_json()
.to_html()
.to_sql()
.to_pickle()
Ainda há mais tipos de arquivos nos quais você pode gravar, portanto, esta lista não é exaustiva.
Observação: Para encontrar métodos semelhantes, verifique a documentação oficial sobre serialização, IO e conversão relacionada a
Series
e DataFrame
objetos. Esses métodos têm parâmetros que especificam o caminho do arquivo de destino no qual você salvou os dados e os rótulos. Isso é obrigatório em alguns casos e opcional em outros. Se esta opção estiver disponível e você optar por omiti-la, os métodos retornarão os objetos (como strings ou iteráveis) com o conteúdo de
DataFrame
instâncias. O parâmetro opcional
compression
decide como compactar o arquivo com os dados e rótulos. Você aprenderá mais sobre isso mais adiante. Existem alguns outros parâmetros, mas eles são principalmente específicos para um ou vários métodos. Você não vai entrar em detalhes aqui. Ler arquivos
As funções do Pandas para ler o conteúdo dos arquivos são nomeadas usando o padrão
.read_<file-type>()
, onde <file-type>
indica o tipo de arquivo a ser lido. Você já viu os Pandas read_csv()
e read_excel()
funções. Aqui estão alguns outros:read_json()
read_html()
read_sql()
read_pickle()
Essas funções têm um parâmetro que especifica o caminho do arquivo de destino. Pode ser qualquer string válida que represente o caminho, seja em uma máquina local ou em uma URL. Outros objetos também são aceitáveis dependendo do tipo de arquivo.
O parâmetro opcional
compression
determina o tipo de descompactação a ser usado para os arquivos compactados. Você aprenderá sobre isso mais tarde neste tutorial. Existem outros parâmetros, mas são específicos para uma ou várias funções. Você não vai entrar em detalhes aqui. Trabalhando com diferentes tipos de arquivo
A biblioteca Pandas oferece uma ampla gama de possibilidades para salvar seus dados em arquivos e carregar dados de arquivos. Nesta seção, você aprenderá mais sobre como trabalhar com arquivos CSV e Excel. Você também verá como usar outros tipos de arquivos, como JSON, páginas da Web, bancos de dados e arquivos de picles do Python.
Arquivos CSV
Você já aprendeu a ler e escrever arquivos CSV. Agora vamos nos aprofundar um pouco nos detalhes. Quando você usa
.to_csv()
para salvar seu DataFrame
, você pode fornecer um argumento para o parâmetro path_or_buf
para especificar o caminho, nome e extensão do arquivo de destino. path_or_buf
é o primeiro argumento .to_csv()
terá. Pode ser qualquer string que represente um caminho de arquivo válido que inclua o nome do arquivo e sua extensão. Você já viu isso em um exemplo anterior. No entanto, se você omitir path_or_buf
, então .to_csv()
não criará nenhum arquivo. Em vez disso, ele retornará a string correspondente:>>>
>>> df = pd.DataFrame(data=data).T
>>> s = df.to_csv()
>>> print(s)
,COUNTRY,POP,AREA,GDP,CONT,IND_DAY
CHN,China,1398.72,9596.96,12234.78,Asia,
IND,India,1351.16,3287.26,2575.67,Asia,1947-08-15
USA,US,329.74,9833.52,19485.39,N.America,1776-07-04
IDN,Indonesia,268.07,1910.93,1015.54,Asia,1945-08-17
BRA,Brazil,210.32,8515.77,2055.51,S.America,1822-09-07
PAK,Pakistan,205.71,881.91,302.14,Asia,1947-08-14
NGA,Nigeria,200.96,923.77,375.77,Africa,1960-10-01
BGD,Bangladesh,167.09,147.57,245.63,Asia,1971-03-26
RUS,Russia,146.79,17098.25,1530.75,,1992-06-12
MEX,Mexico,126.58,1964.38,1158.23,N.America,1810-09-16
JPN,Japan,126.22,377.97,4872.42,Asia,
DEU,Germany,83.02,357.11,3693.2,Europe,
FRA,France,67.02,640.68,2582.49,Europe,1789-07-14
GBR,UK,66.44,242.5,2631.23,Europe,
ITA,Italy,60.36,301.34,1943.84,Europe,
ARG,Argentina,44.94,2780.4,637.49,S.America,1816-07-09
DZA,Algeria,43.38,2381.74,167.56,Africa,1962-07-05
CAN,Canada,37.59,9984.67,1647.12,N.America,1867-07-01
AUS,Australia,25.47,7692.02,1408.68,Oceania,
KAZ,Kazakhstan,18.53,2724.9,159.41,Asia,1991-12-16
Agora você tem a string
s
em vez de um arquivo CSV. Você também tem alguns valores ausentes em seu DataFrame
objeto. Por exemplo, o continente para a Rússia e os dias de independência para vários países (China, Japão e assim por diante) não estão disponíveis. Em ciência de dados e aprendizado de máquina, você deve lidar com os valores ausentes com cuidado. Pandas se destaca aqui! Por padrão, o Pandas usa o valor NaN para substituir os valores ausentes. Observação:
nan
, que significa “not a number”, é um valor de ponto flutuante específico em Python. Você pode obter um
nan
valor com qualquer uma das seguintes funções:float('nan')
math.nan
numpy.nan
O continente que corresponde à Rússia em
df
é nan
:>>>
>>> df.loc['RUS', 'CONT']
nan
Este exemplo usa
.loc[]
para obter dados com os nomes de linha e coluna especificados. Quando você salva seu
DataFrame
para um arquivo CSV, strings vazias (''
) representará os dados ausentes. Você pode ver isso em seu arquivo data.csv
e na string s
. Se você deseja alterar esse comportamento, use o parâmetro opcional na_rep
:>>>
>>> df.to_csv('new-data.csv', na_rep='(missing)')
Este código produz o arquivo
new-data.csv
onde os valores ausentes não são mais strings vazias. Você pode expandir o bloco de código abaixo para ver como esse arquivo deve ficar:,COUNTRY,POP,AREA,GDP,CONT,IND_DAY
CHN,China,1398.72,9596.96,12234.78,Asia,(missing)
IND,India,1351.16,3287.26,2575.67,Asia,1947-08-15
USA,US,329.74,9833.52,19485.39,N.America,1776-07-04
IDN,Indonesia,268.07,1910.93,1015.54,Asia,1945-08-17
BRA,Brazil,210.32,8515.77,2055.51,S.America,1822-09-07
PAK,Pakistan,205.71,881.91,302.14,Asia,1947-08-14
NGA,Nigeria,200.96,923.77,375.77,Africa,1960-10-01
BGD,Bangladesh,167.09,147.57,245.63,Asia,1971-03-26
RUS,Russia,146.79,17098.25,1530.75,(missing),1992-06-12
MEX,Mexico,126.58,1964.38,1158.23,N.America,1810-09-16
JPN,Japan,126.22,377.97,4872.42,Asia,(missing)
DEU,Germany,83.02,357.11,3693.2,Europe,(missing)
FRA,France,67.02,640.68,2582.49,Europe,1789-07-14
GBR,UK,66.44,242.5,2631.23,Europe,(missing)
ITA,Italy,60.36,301.34,1943.84,Europe,(missing)
ARG,Argentina,44.94,2780.4,637.49,S.America,1816-07-09
DZA,Algeria,43.38,2381.74,167.56,Africa,1962-07-05
CAN,Canada,37.59,9984.67,1647.12,N.America,1867-07-01
AUS,Australia,25.47,7692.02,1408.68,Oceania,(missing)
KAZ,Kazakhstan,18.53,2724.9,159.41,Asia,1991-12-16
Agora, a string
'(missing)'
no arquivo corresponde ao nan
valores de df
. Quando o Pandas lê arquivos, ele considera a string vazia (
''
) and a few others as missing values by default:'nan'
'-nan'
'NA'
'N/A'
'NaN'
'null'
If you don’t want this behavior, then you can pass
keep_default_na=False
to the Pandas read_csv()
função. To specify other labels for missing values, use the parameter na_values
:>>>
>>> pd.read_csv('new-data.csv', index_col=0, na_values='(missing)')
COUNTRY POP AREA GDP CONT IND_DAY
CHN China 1398.72 9596.96 12234.78 Asia NaN
IND India 1351.16 3287.26 2575.67 Asia 1947-08-15
USA US 329.74 9833.52 19485.39 N.America 1776-07-04
IDN Indonesia 268.07 1910.93 1015.54 Asia 1945-08-17
BRA Brazil 210.32 8515.77 2055.51 S.America 1822-09-07
PAK Pakistan 205.71 881.91 302.14 Asia 1947-08-14
NGA Nigeria 200.96 923.77 375.77 Africa 1960-10-01
BGD Bangladesh 167.09 147.57 245.63 Asia 1971-03-26
RUS Russia 146.79 17098.25 1530.75 NaN 1992-06-12
MEX Mexico 126.58 1964.38 1158.23 N.America 1810-09-16
JPN Japan 126.22 377.97 4872.42 Asia NaN
DEU Germany 83.02 357.11 3693.20 Europe NaN
FRA France 67.02 640.68 2582.49 Europe 1789-07-14
GBR UK 66.44 242.50 2631.23 Europe NaN
ITA Italy 60.36 301.34 1943.84 Europe NaN
ARG Argentina 44.94 2780.40 637.49 S.America 1816-07-09
DZA Algeria 43.38 2381.74 167.56 Africa 1962-07-05
CAN Canada 37.59 9984.67 1647.12 N.America 1867-07-01
AUS Australia 25.47 7692.02 1408.68 Oceania NaN
KAZ Kazakhstan 18.53 2724.90 159.41 Asia 1991-12-16
Here, you’ve marked the string
'(missing)'
as a new missing data label, and Pandas replaced it with nan
when it read the file. When you load data from a file, Pandas assigns the data types to the values of each column by default. You can check these types with
.dtypes
:>>>
>>> df = pd.read_csv('data.csv', index_col=0)
>>> df.dtypes
COUNTRY object
POP float64
AREA float64
GDP float64
CONT object
IND_DAY object
dtype: object
The columns with strings and dates (
'COUNTRY'
, 'CONT'
, and 'IND_DAY'
) have the data type object
. Meanwhile, the numeric columns contain 64-bit floating-point numbers (float64
). You can use the parameter
dtype
to specify the desired data types and parse_dates
to force use of datetimes:>>>
>>> dtypes = {'POP': 'float32', 'AREA': 'float32', 'GDP': 'float32'}
>>> df = pd.read_csv('data.csv', index_col=0, dtype=dtypes,
... parse_dates=['IND_DAY'])
>>> df.dtypes
COUNTRY object
POP float32
AREA float32
GDP float32
CONT object
IND_DAY datetime64[ns]
dtype: object
>>> df['IND_DAY']
CHN NaT
IND 1947-08-15
USA 1776-07-04
IDN 1945-08-17
BRA 1822-09-07
PAK 1947-08-14
NGA 1960-10-01
BGD 1971-03-26
RUS 1992-06-12
MEX 1810-09-16
JPN NaT
DEU NaT
FRA 1789-07-14
GBR NaT
ITA NaT
ARG 1816-07-09
DZA 1962-07-05
CAN 1867-07-01
AUS NaT
KAZ 1991-12-16
Name: IND_DAY, dtype: datetime64[ns]
Now, you have 32-bit floating-point numbers (
float32
) as specified with dtype
. These differ slightly from the original 64-bit numbers because of smaller precision . The values in the last column are considered as dates and have the data type datetime64
. That’s why the NaN
values in this column are replaced with NaT
. Now that you have real dates, you can save them in the format you like:
>>>
>>> df = pd.read_csv('data.csv', index_col=0, parse_dates=['IND_DAY'])
>>> df.to_csv('formatted-data.csv', date_format='%B %d, %Y')
Here, you’ve specified the parameter
date_format
to be '%B %d, %Y'
. You can expand the code block below to see the resulting file:,COUNTRY,POP,AREA,GDP,CONT,IND_DAY
CHN,China,1398.72,9596.96,12234.78,Asia,
IND,India,1351.16,3287.26,2575.67,Asia,"August 15, 1947"
USA,US,329.74,9833.52,19485.39,N.America,"July 04, 1776"
IDN,Indonesia,268.07,1910.93,1015.54,Asia,"August 17, 1945"
BRA,Brazil,210.32,8515.77,2055.51,S.America,"September 07, 1822"
PAK,Pakistan,205.71,881.91,302.14,Asia,"August 14, 1947"
NGA,Nigeria,200.96,923.77,375.77,Africa,"October 01, 1960"
BGD,Bangladesh,167.09,147.57,245.63,Asia,"March 26, 1971"
RUS,Russia,146.79,17098.25,1530.75,,"June 12, 1992"
MEX,Mexico,126.58,1964.38,1158.23,N.America,"September 16, 1810"
JPN,Japan,126.22,377.97,4872.42,Asia,
DEU,Germany,83.02,357.11,3693.2,Europe,
FRA,France,67.02,640.68,2582.49,Europe,"July 14, 1789"
GBR,UK,66.44,242.5,2631.23,Europe,
ITA,Italy,60.36,301.34,1943.84,Europe,
ARG,Argentina,44.94,2780.4,637.49,S.America,"July 09, 1816"
DZA,Algeria,43.38,2381.74,167.56,Africa,"July 05, 1962"
CAN,Canada,37.59,9984.67,1647.12,N.America,"July 01, 1867"
AUS,Australia,25.47,7692.02,1408.68,Oceania,
KAZ,Kazakhstan,18.53,2724.9,159.41,Asia,"December 16, 1991"
The format of the dates is different now. The format
'%B %d, %Y'
means the date will first display the full name of the month, then the day followed by a comma, and finally the full year. There are several other optional parameters that you can use with
.to_csv()
:sep
denotes a values separator.decimal
indicates a decimal separator.encoding
sets the file encoding.header
specifies whether you want to write column labels in the file.
Here’s how you would pass arguments for
sep
and header
:>>>
>>> s = df.to_csv(sep=';', header=False)
>>> print(s)
CHN;China;1398.72;9596.96;12234.78;Asia;
IND;India;1351.16;3287.26;2575.67;Asia;1947-08-15
USA;US;329.74;9833.52;19485.39;N.America;1776-07-04
IDN;Indonesia;268.07;1910.93;1015.54;Asia;1945-08-17
BRA;Brazil;210.32;8515.77;2055.51;S.America;1822-09-07
PAK;Pakistan;205.71;881.91;302.14;Asia;1947-08-14
NGA;Nigeria;200.96;923.77;375.77;Africa;1960-10-01
BGD;Bangladesh;167.09;147.57;245.63;Asia;1971-03-26
RUS;Russia;146.79;17098.25;1530.75;;1992-06-12
MEX;Mexico;126.58;1964.38;1158.23;N.America;1810-09-16
JPN;Japan;126.22;377.97;4872.42;Asia;
DEU;Germany;83.02;357.11;3693.2;Europe;
FRA;France;67.02;640.68;2582.49;Europe;1789-07-14
GBR;UK;66.44;242.5;2631.23;Europe;
ITA;Italy;60.36;301.34;1943.84;Europe;
ARG;Argentina;44.94;2780.4;637.49;S.America;1816-07-09
DZA;Algeria;43.38;2381.74;167.56;Africa;1962-07-05
CAN;Canada;37.59;9984.67;1647.12;N.America;1867-07-01
AUS;Australia;25.47;7692.02;1408.68;Oceania;
KAZ;Kazakhstan;18.53;2724.9;159.41;Asia;1991-12-16
The data is separated with a semicolon (
';'
) because you’ve specified sep=';'
. Also, since you passed header=False
, you see your data without the header row of column names. The Pandas
read_csv()
function has many additional options for managing missing data, working with dates and times, quoting, encoding, handling errors, and more. For instance, if you have a file with one data column and want to get a Series
object instead of a DataFrame
, then you can pass squeeze=True
to read_csv()
. You’ll learn later on about data compression and decompression, as well as how to skip rows and columns. JSON Files
JSON stands for JavaScript object notation. JSON files are plaintext files used for data interchange, and humans can read them easily. They follow the ISO/IEC 21778:2017 and ECMA-404 standards and use the
.json
extensão. Python and Pandas work well with JSON files, as Python’s json library offers built-in support for them. You can save the data from your
DataFrame
to a JSON file with .to_json()
. Start by creating a DataFrame
object again. Use the dictionary data
that holds the data about countries and then apply .to_json()
:>>>
>>> df = pd.DataFrame(data=data).T
>>> df.to_json('data-columns.json')
This code produces the file
data-columns.json
. You can expand the code block below to see how this file should look:{"COUNTRY":{"CHN":"China","IND":"India","USA":"US","IDN":"Indonesia","BRA":"Brazil","PAK":"Pakistan","NGA":"Nigeria","BGD":"Bangladesh","RUS":"Russia","MEX":"Mexico","JPN":"Japan","DEU":"Germany","FRA":"France","GBR":"UK","ITA":"Italy","ARG":"Argentina","DZA":"Algeria","CAN":"Canada","AUS":"Australia","KAZ":"Kazakhstan"},"POP":{"CHN":1398.72,"IND":1351.16,"USA":329.74,"IDN":268.07,"BRA":210.32,"PAK":205.71,"NGA":200.96,"BGD":167.09,"RUS":146.79,"MEX":126.58,"JPN":126.22,"DEU":83.02,"FRA":67.02,"GBR":66.44,"ITA":60.36,"ARG":44.94,"DZA":43.38,"CAN":37.59,"AUS":25.47,"KAZ":18.53},"AREA":{"CHN":9596.96,"IND":3287.26,"USA":9833.52,"IDN":1910.93,"BRA":8515.77,"PAK":881.91,"NGA":923.77,"BGD":147.57,"RUS":17098.25,"MEX":1964.38,"JPN":377.97,"DEU":357.11,"FRA":640.68,"GBR":242.5,"ITA":301.34,"ARG":2780.4,"DZA":2381.74,"CAN":9984.67,"AUS":7692.02,"KAZ":2724.9},"GDP":{"CHN":12234.78,"IND":2575.67,"USA":19485.39,"IDN":1015.54,"BRA":2055.51,"PAK":302.14,"NGA":375.77,"BGD":245.63,"RUS":1530.75,"MEX":1158.23,"JPN":4872.42,"DEU":3693.2,"FRA":2582.49,"GBR":2631.23,"ITA":1943.84,"ARG":637.49,"DZA":167.56,"CAN":1647.12,"AUS":1408.68,"KAZ":159.41},"CONT":{"CHN":"Asia","IND":"Asia","USA":"N.America","IDN":"Asia","BRA":"S.America","PAK":"Asia","NGA":"Africa","BGD":"Asia","RUS":null,"MEX":"N.America","JPN":"Asia","DEU":"Europe","FRA":"Europe","GBR":"Europe","ITA":"Europe","ARG":"S.America","DZA":"Africa","CAN":"N.America","AUS":"Oceania","KAZ":"Asia"},"IND_DAY":{"CHN":null,"IND":"1947-08-15","USA":"1776-07-04","IDN":"1945-08-17","BRA":"1822-09-07","PAK":"1947-08-14","NGA":"1960-10-01","BGD":"1971-03-26","RUS":"1992-06-12","MEX":"1810-09-16","JPN":null,"DEU":null,"FRA":"1789-07-14","GBR":null,"ITA":null,"ARG":"1816-07-09","DZA":"1962-07-05","CAN":"1867-07-01","AUS":null,"KAZ":"1991-12-16"}}
data-columns.json
has one large dictionary with the column labels as keys and the corresponding inner dictionaries as values. You can get a different file structure if you pass an argument for the optional parameter
orient
:>>>
>>> df.to_json('data-index.json', orient='index')
The
orient
parameter defaults to 'columns'
. Here, you’ve set it to index
. You should get a new file
data-index.json
. You can expand the code block below to see the changes:{"CHN":{"COUNTRY":"China","POP":1398.72,"AREA":9596.96,"GDP":12234.78,"CONT":"Asia","IND_DAY":null},"IND":{"COUNTRY":"India","POP":1351.16,"AREA":3287.26,"GDP":2575.67,"CONT":"Asia","IND_DAY":"1947-08-15"},"USA":{"COUNTRY":"US","POP":329.74,"AREA":9833.52,"GDP":19485.39,"CONT":"N.America","IND_DAY":"1776-07-04"},"IDN":{"COUNTRY":"Indonesia","POP":268.07,"AREA":1910.93,"GDP":1015.54,"CONT":"Asia","IND_DAY":"1945-08-17"},"BRA":{"COUNTRY":"Brazil","POP":210.32,"AREA":8515.77,"GDP":2055.51,"CONT":"S.America","IND_DAY":"1822-09-07"},"PAK":{"COUNTRY":"Pakistan","POP":205.71,"AREA":881.91,"GDP":302.14,"CONT":"Asia","IND_DAY":"1947-08-14"},"NGA":{"COUNTRY":"Nigeria","POP":200.96,"AREA":923.77,"GDP":375.77,"CONT":"Africa","IND_DAY":"1960-10-01"},"BGD":{"COUNTRY":"Bangladesh","POP":167.09,"AREA":147.57,"GDP":245.63,"CONT":"Asia","IND_DAY":"1971-03-26"},"RUS":{"COUNTRY":"Russia","POP":146.79,"AREA":17098.25,"GDP":1530.75,"CONT":null,"IND_DAY":"1992-06-12"},"MEX":{"COUNTRY":"Mexico","POP":126.58,"AREA":1964.38,"GDP":1158.23,"CONT":"N.America","IND_DAY":"1810-09-16"},"JPN":{"COUNTRY":"Japan","POP":126.22,"AREA":377.97,"GDP":4872.42,"CONT":"Asia","IND_DAY":null},"DEU":{"COUNTRY":"Germany","POP":83.02,"AREA":357.11,"GDP":3693.2,"CONT":"Europe","IND_DAY":null},"FRA":{"COUNTRY":"France","POP":67.02,"AREA":640.68,"GDP":2582.49,"CONT":"Europe","IND_DAY":"1789-07-14"},"GBR":{"COUNTRY":"UK","POP":66.44,"AREA":242.5,"GDP":2631.23,"CONT":"Europe","IND_DAY":null},"ITA":{"COUNTRY":"Italy","POP":60.36,"AREA":301.34,"GDP":1943.84,"CONT":"Europe","IND_DAY":null},"ARG":{"COUNTRY":"Argentina","POP":44.94,"AREA":2780.4,"GDP":637.49,"CONT":"S.America","IND_DAY":"1816-07-09"},"DZA":{"COUNTRY":"Algeria","POP":43.38,"AREA":2381.74,"GDP":167.56,"CONT":"Africa","IND_DAY":"1962-07-05"},"CAN":{"COUNTRY":"Canada","POP":37.59,"AREA":9984.67,"GDP":1647.12,"CONT":"N.America","IND_DAY":"1867-07-01"},"AUS":{"COUNTRY":"Australia","POP":25.47,"AREA":7692.02,"GDP":1408.68,"CONT":"Oceania","IND_DAY":null},"KAZ":{"COUNTRY":"Kazakhstan","POP":18.53,"AREA":2724.9,"GDP":159.41,"CONT":"Asia","IND_DAY":"1991-12-16"}}
data-index.json
also has one large dictionary, but this time the row labels are the keys, and the inner dictionaries are the values. There are few more options for
orient
. One of them is 'records'
:>>>
>>> df.to_json('data-records.json', orient='records')
This code should yield the file
data-records.json
. You can expand the code block below to see the content:[{"COUNTRY":"China","POP":1398.72,"AREA":9596.96,"GDP":12234.78,"CONT":"Asia","IND_DAY":null},{"COUNTRY":"India","POP":1351.16,"AREA":3287.26,"GDP":2575.67,"CONT":"Asia","IND_DAY":"1947-08-15"},{"COUNTRY":"US","POP":329.74,"AREA":9833.52,"GDP":19485.39,"CONT":"N.America","IND_DAY":"1776-07-04"},{"COUNTRY":"Indonesia","POP":268.07,"AREA":1910.93,"GDP":1015.54,"CONT":"Asia","IND_DAY":"1945-08-17"},{"COUNTRY":"Brazil","POP":210.32,"AREA":8515.77,"GDP":2055.51,"CONT":"S.America","IND_DAY":"1822-09-07"},{"COUNTRY":"Pakistan","POP":205.71,"AREA":881.91,"GDP":302.14,"CONT":"Asia","IND_DAY":"1947-08-14"},{"COUNTRY":"Nigeria","POP":200.96,"AREA":923.77,"GDP":375.77,"CONT":"Africa","IND_DAY":"1960-10-01"},{"COUNTRY":"Bangladesh","POP":167.09,"AREA":147.57,"GDP":245.63,"CONT":"Asia","IND_DAY":"1971-03-26"},{"COUNTRY":"Russia","POP":146.79,"AREA":17098.25,"GDP":1530.75,"CONT":null,"IND_DAY":"1992-06-12"},{"COUNTRY":"Mexico","POP":126.58,"AREA":1964.38,"GDP":1158.23,"CONT":"N.America","IND_DAY":"1810-09-16"},{"COUNTRY":"Japan","POP":126.22,"AREA":377.97,"GDP":4872.42,"CONT":"Asia","IND_DAY":null},{"COUNTRY":"Germany","POP":83.02,"AREA":357.11,"GDP":3693.2,"CONT":"Europe","IND_DAY":null},{"COUNTRY":"France","POP":67.02,"AREA":640.68,"GDP":2582.49,"CONT":"Europe","IND_DAY":"1789-07-14"},{"COUNTRY":"UK","POP":66.44,"AREA":242.5,"GDP":2631.23,"CONT":"Europe","IND_DAY":null},{"COUNTRY":"Italy","POP":60.36,"AREA":301.34,"GDP":1943.84,"CONT":"Europe","IND_DAY":null},{"COUNTRY":"Argentina","POP":44.94,"AREA":2780.4,"GDP":637.49,"CONT":"S.America","IND_DAY":"1816-07-09"},{"COUNTRY":"Algeria","POP":43.38,"AREA":2381.74,"GDP":167.56,"CONT":"Africa","IND_DAY":"1962-07-05"},{"COUNTRY":"Canada","POP":37.59,"AREA":9984.67,"GDP":1647.12,"CONT":"N.America","IND_DAY":"1867-07-01"},{"COUNTRY":"Australia","POP":25.47,"AREA":7692.02,"GDP":1408.68,"CONT":"Oceania","IND_DAY":null},{"COUNTRY":"Kazakhstan","POP":18.53,"AREA":2724.9,"GDP":159.41,"CONT":"Asia","IND_DAY":"1991-12-16"}]
data-records.json
holds a list with one dictionary for each row. The row labels are not written. You can get another interesting file structure with
orient='split'
:>>>
>>> df.to_json('data-split.json', orient='split')
The resulting file is
data-split.json
. You can expand the code block below to see how this file should look:{"columns":["COUNTRY","POP","AREA","GDP","CONT","IND_DAY"],"index":["CHN","IND","USA","IDN","BRA","PAK","NGA","BGD","RUS","MEX","JPN","DEU","FRA","GBR","ITA","ARG","DZA","CAN","AUS","KAZ"],"data":[["China",1398.72,9596.96,12234.78,"Asia",null],["India",1351.16,3287.26,2575.67,"Asia","1947-08-15"],["US",329.74,9833.52,19485.39,"N.America","1776-07-04"],["Indonesia",268.07,1910.93,1015.54,"Asia","1945-08-17"],["Brazil",210.32,8515.77,2055.51,"S.America","1822-09-07"],["Pakistan",205.71,881.91,302.14,"Asia","1947-08-14"],["Nigeria",200.96,923.77,375.77,"Africa","1960-10-01"],["Bangladesh",167.09,147.57,245.63,"Asia","1971-03-26"],["Russia",146.79,17098.25,1530.75,null,"1992-06-12"],["Mexico",126.58,1964.38,1158.23,"N.America","1810-09-16"],["Japan",126.22,377.97,4872.42,"Asia",null],["Germany",83.02,357.11,3693.2,"Europe",null],["France",67.02,640.68,2582.49,"Europe","1789-07-14"],["UK",66.44,242.5,2631.23,"Europe",null],["Italy",60.36,301.34,1943.84,"Europe",null],["Argentina",44.94,2780.4,637.49,"S.America","1816-07-09"],["Algeria",43.38,2381.74,167.56,"Africa","1962-07-05"],["Canada",37.59,9984.67,1647.12,"N.America","1867-07-01"],["Australia",25.47,7692.02,1408.68,"Oceania",null],["Kazakhstan",18.53,2724.9,159.41,"Asia","1991-12-16"]]}
data-split.json
contains one dictionary that holds the following lists:- The names of the columns
- The labels of the rows
- The inner lists (two-dimensional sequence) that hold data values
If you don’t provide the value for the optional parameter
path_or_buf
that defines the file path, then .to_json()
will return a JSON string instead of writing the results to a file. This behavior is consistent with .to_csv()
. There are other optional parameters you can use. For instance, you can set
index=False
to forgo saving row labels. You can manipulate precision with double_precision
, and dates with date_format
and date_unit
. These last two parameters are particularly important when you have time series among your data:>>>
>>> df = pd.DataFrame(data=data).T
>>> df['IND_DAY'] = pd.to_datetime(df['IND_DAY'])
>>> df.dtypes
COUNTRY object
POP object
AREA object
GDP object
CONT object
IND_DAY datetime64[ns]
dtype: object
>>> df.to_json('data-time.json')
In this example, you’ve created the
DataFrame
from the dictionary data
and used to_datetime()
to convert the values in the last column to datetime64
. You can expand the code block below to see the resulting file:{"COUNTRY":{"CHN":"China","IND":"India","USA":"US","IDN":"Indonesia","BRA":"Brazil","PAK":"Pakistan","NGA":"Nigeria","BGD":"Bangladesh","RUS":"Russia","MEX":"Mexico","JPN":"Japan","DEU":"Germany","FRA":"France","GBR":"UK","ITA":"Italy","ARG":"Argentina","DZA":"Algeria","CAN":"Canada","AUS":"Australia","KAZ":"Kazakhstan"},"POP":{"CHN":1398.72,"IND":1351.16,"USA":329.74,"IDN":268.07,"BRA":210.32,"PAK":205.71,"NGA":200.96,"BGD":167.09,"RUS":146.79,"MEX":126.58,"JPN":126.22,"DEU":83.02,"FRA":67.02,"GBR":66.44,"ITA":60.36,"ARG":44.94,"DZA":43.38,"CAN":37.59,"AUS":25.47,"KAZ":18.53},"AREA":{"CHN":9596.96,"IND":3287.26,"USA":9833.52,"IDN":1910.93,"BRA":8515.77,"PAK":881.91,"NGA":923.77,"BGD":147.57,"RUS":17098.25,"MEX":1964.38,"JPN":377.97,"DEU":357.11,"FRA":640.68,"GBR":242.5,"ITA":301.34,"ARG":2780.4,"DZA":2381.74,"CAN":9984.67,"AUS":7692.02,"KAZ":2724.9},"GDP":{"CHN":12234.78,"IND":2575.67,"USA":19485.39,"IDN":1015.54,"BRA":2055.51,"PAK":302.14,"NGA":375.77,"BGD":245.63,"RUS":1530.75,"MEX":1158.23,"JPN":4872.42,"DEU":3693.2,"FRA":2582.49,"GBR":2631.23,"ITA":1943.84,"ARG":637.49,"DZA":167.56,"CAN":1647.12,"AUS":1408.68,"KAZ":159.41},"CONT":{"CHN":"Asia","IND":"Asia","USA":"N.America","IDN":"Asia","BRA":"S.America","PAK":"Asia","NGA":"Africa","BGD":"Asia","RUS":null,"MEX":"N.America","JPN":"Asia","DEU":"Europe","FRA":"Europe","GBR":"Europe","ITA":"Europe","ARG":"S.America","DZA":"Africa","CAN":"N.America","AUS":"Oceania","KAZ":"Asia"},"IND_DAY":{"CHN":null,"IND":-706320000000,"USA":-6106060800000,"IDN":-769219200000,"BRA":-4648924800000,"PAK":-706406400000,"NGA":-291945600000,"BGD":38793600000,"RUS":708307200000,"MEX":-5026838400000,"JPN":null,"DEU":null,"FRA":-5694969600000,"GBR":null,"ITA":null,"ARG":-4843411200000,"DZA":-236476800000,"CAN":-3234729600000,"AUS":null,"KAZ":692841600000}}
In this file, you have large integers instead of dates for the independence days. That’s because the default value of the optional parameter
date_format
is 'epoch'
whenever orient
isn’t 'table'
. This default behavior expresses dates as an epoch in milliseconds relative to midnight on January 1, 1970. However, if you pass
date_format='iso'
, then you’ll get the dates in the ISO 8601 format. In addition, date_unit
decides the units of time:>>>
>>> df = pd.DataFrame(data=data).T
>>> df['IND_DAY'] = pd.to_datetime(df['IND_DAY'])
>>> df.to_json('new-data-time.json', date_format='iso', date_unit='s')
This code produces the following JSON file:
{"COUNTRY":{"CHN":"China","IND":"India","USA":"US","IDN":"Indonesia","BRA":"Brazil","PAK":"Pakistan","NGA":"Nigeria","BGD":"Bangladesh","RUS":"Russia","MEX":"Mexico","JPN":"Japan","DEU":"Germany","FRA":"France","GBR":"UK","ITA":"Italy","ARG":"Argentina","DZA":"Algeria","CAN":"Canada","AUS":"Australia","KAZ":"Kazakhstan"},"POP":{"CHN":1398.72,"IND":1351.16,"USA":329.74,"IDN":268.07,"BRA":210.32,"PAK":205.71,"NGA":200.96,"BGD":167.09,"RUS":146.79,"MEX":126.58,"JPN":126.22,"DEU":83.02,"FRA":67.02,"GBR":66.44,"ITA":60.36,"ARG":44.94,"DZA":43.38,"CAN":37.59,"AUS":25.47,"KAZ":18.53},"AREA":{"CHN":9596.96,"IND":3287.26,"USA":9833.52,"IDN":1910.93,"BRA":8515.77,"PAK":881.91,"NGA":923.77,"BGD":147.57,"RUS":17098.25,"MEX":1964.38,"JPN":377.97,"DEU":357.11,"FRA":640.68,"GBR":242.5,"ITA":301.34,"ARG":2780.4,"DZA":2381.74,"CAN":9984.67,"AUS":7692.02,"KAZ":2724.9},"GDP":{"CHN":12234.78,"IND":2575.67,"USA":19485.39,"IDN":1015.54,"BRA":2055.51,"PAK":302.14,"NGA":375.77,"BGD":245.63,"RUS":1530.75,"MEX":1158.23,"JPN":4872.42,"DEU":3693.2,"FRA":2582.49,"GBR":2631.23,"ITA":1943.84,"ARG":637.49,"DZA":167.56,"CAN":1647.12,"AUS":1408.68,"KAZ":159.41},"CONT":{"CHN":"Asia","IND":"Asia","USA":"N.America","IDN":"Asia","BRA":"S.America","PAK":"Asia","NGA":"Africa","BGD":"Asia","RUS":null,"MEX":"N.America","JPN":"Asia","DEU":"Europe","FRA":"Europe","GBR":"Europe","ITA":"Europe","ARG":"S.America","DZA":"Africa","CAN":"N.America","AUS":"Oceania","KAZ":"Asia"},"IND_DAY":{"CHN":null,"IND":"1947-08-15T00:00:00Z","USA":"1776-07-04T00:00:00Z","IDN":"1945-08-17T00:00:00Z","BRA":"1822-09-07T00:00:00Z","PAK":"1947-08-14T00:00:00Z","NGA":"1960-10-01T00:00:00Z","BGD":"1971-03-26T00:00:00Z","RUS":"1992-06-12T00:00:00Z","MEX":"1810-09-16T00:00:00Z","JPN":null,"DEU":null,"FRA":"1789-07-14T00:00:00Z","GBR":null,"ITA":null,"ARG":"1816-07-09T00:00:00Z","DZA":"1962-07-05T00:00:00Z","CAN":"1867-07-01T00:00:00Z","AUS":null,"KAZ":"1991-12-16T00:00:00Z"}}
The dates in the resulting file are in the ISO 8601 format.
You can load the data from a JSON file with
read_json()
:>>>
>>> df = pd.read_json('data-index.json', orient='index',
... convert_dates=['IND_DAY'])
The parameter
convert_dates
has a similar purpose as parse_dates
when you use it to read CSV files. The optional parameter orient
is very important because it specifies how Pandas understands the structure of the file. There are other optional parameters you can use as well:
- Set the encoding with
encoding
. - Manipulate dates with
convert_dates
andkeep_default_dates
. - Impact precision with
dtype
andprecise_float
. - Decode numeric data directly to NumPy arrays with
numpy=True
.
Note that you might lose the order of rows and columns when using the JSON format to store your data.
HTML Files
An HTML is a plaintext file that uses hypertext markup language to help browsers render web pages. The extensions for HTML files are
.html
and .htm
. You’ll need to install an HTML parser library like lxml or html5lib to be able to work with HTML files:$pip install lxml html5lib
You can also use Conda to install the same packages:
$ conda install lxml html5lib
Once you have these libraries, you can save the contents of your
DataFrame
as an HTML file with .to_html()
:>>>
df = pd.DataFrame(data=data).T
df.to_html('data.html')
This code generates a file
data.html
. You can expand the code block below to see how this file should look:<table border="1" class="dataframe">
<thead>
<tr style="text-align: right;">
<th></th>
<th>COUNTRY</th>
<th>POP</th>
<th>AREA</th>
<th>GDP</th>
<th>CONT</th>
<th>IND_DAY</th>
</tr>
</thead>
<tbody>
<tr>
<th>CHN</th>
<td>China</td>
<td>1398.72</td>
<td>9596.96</td>
<td>12234.8</td>
<td>Asia</td>
<td>NaN</td>
</tr>
<tr>
<th>IND</th>
<td>India</td>
<td>1351.16</td>
<td>3287.26</td>
<td>2575.67</td>
<td>Asia</td>
<td>1947-08-15</td>
</tr>
<tr>
<th>USA</th>
<td>US</td>
<td>329.74</td>
<td>9833.52</td>
<td>19485.4</td>
<td>N.America</td>
<td>1776-07-04</td>
</tr>
<tr>
<th>IDN</th>
<td>Indonesia</td>
<td>268.07</td>
<td>1910.93</td>
<td>1015.54</td>
<td>Asia</td>
<td>1945-08-17</td>
</tr>
<tr>
<th>BRA</th>
<td>Brazil</td>
<td>210.32</td>
<td>8515.77</td>
<td>2055.51</td>
<td>S.America</td>
<td>1822-09-07</td>
</tr>
<tr>
<th>PAK</th>
<td>Pakistan</td>
<td>205.71</td>
<td>881.91</td>
<td>302.14</td>
<td>Asia</td>
<td>1947-08-14</td>
</tr>
<tr>
<th>NGA</th>
<td>Nigeria</td>
<td>200.96</td>
<td>923.77</td>
<td>375.77</td>
<td>Africa</td>
<td>1960-10-01</td>
</tr>
<tr>
<th>BGD</th>
<td>Bangladesh</td>
<td>167.09</td>
<td>147.57</td>
<td>245.63</td>
<td>Asia</td>
<td>1971-03-26</td>
</tr>
<tr>
<th>RUS</th>
<td>Russia</td>
<td>146.79</td>
<td>17098.2</td>
<td>1530.75</td>
<td>NaN</td>
<td>1992-06-12</td>
</tr>
<tr>
<th>MEX</th>
<td>Mexico</td>
<td>126.58</td>
<td>1964.38</td>
<td>1158.23</td>
<td>N.America</td>
<td>1810-09-16</td>
</tr>
<tr>
<th>JPN</th>
<td>Japan</td>
<td>126.22</td>
<td>377.97</td>
<td>4872.42</td>
<td>Asia</td>
<td>NaN</td>
</tr>
<tr>
<th>DEU</th>
<td>Germany</td>
<td>83.02</td>
<td>357.11</td>
<td>3693.2</td>
<td>Europe</td>
<td>NaN</td>
</tr>
<tr>
<th>FRA</th>
<td>France</td>
<td>67.02</td>
<td>640.68</td>
<td>2582.49</td>
<td>Europe</td>
<td>1789-07-14</td>
</tr>
<tr>
<th>GBR</th>
<td>UK</td>
<td>66.44</td>
<td>242.5</td>
<td>2631.23</td>
<td>Europe</td>
<td>NaN</td>
</tr>
<tr>
<th>ITA</th>
<td>Italy</td>
<td>60.36</td>
<td>301.34</td>
<td>1943.84</td>
<td>Europe</td>
<td>NaN</td>
</tr>
<tr>
<th>ARG</th>
<td>Argentina</td>
<td>44.94</td>
<td>2780.4</td>
<td>637.49</td>
<td>S.America</td>
<td>1816-07-09</td>
</tr>
<tr>
<th>DZA</th>
<td>Algeria</td>
<td>43.38</td>
<td>2381.74</td>
<td>167.56</td>
<td>Africa</td>
<td>1962-07-05</td>
</tr>
<tr>
<th>CAN</th>
<td>Canada</td>
<td>37.59</td>
<td>9984.67</td>
<td>1647.12</td>
<td>N.America</td>
<td>1867-07-01</td>
</tr>
<tr>
<th>AUS</th>
<td>Australia</td>
<td>25.47</td>
<td>7692.02</td>
<td>1408.68</td>
<td>Oceania</td>
<td>NaN</td>
</tr>
<tr>
<th>KAZ</th>
<td>Kazakhstan</td>
<td>18.53</td>
<td>2724.9</td>
<td>159.41</td>
<td>Asia</td>
<td>1991-12-16</td>
</tr>
</tbody>
</table>
This file shows the
DataFrame
contents nicely. However, notice that you haven’t obtained an entire web page. You’ve just output the data that corresponds to df
in the HTML format. .to_html()
won’t create a file if you don’t provide the optional parameter buf
, which denotes the buffer to write to. If you leave this parameter out, then your code will return a string as it did with .to_csv()
and .to_json()
. Here are some other optional parameters:
header
determines whether to save the column names.index
determines whether to save the row labels.classes
assigns cascading style sheet (CSS) classes.render_links
specifies whether to convert URLs to HTML links.table_id
assigns the CSSid
to thetable
tag.escape
decides whether to convert the characters<
,>
, and&
to HTML-safe strings.
You use parameters like these to specify different aspects of the resulting files or strings.
You can create a
DataFrame
object from a suitable HTML file using read_html()
, which will return a DataFrame
instance or a list of them:>>>
>>> df = pd.read_html('data.html', index_col=0, parse_dates=['IND_DAY'])
This is very similar to what you did when reading CSV files. You also have parameters that help you work with dates, missing values, precision, encoding, HTML parsers, and more.
Excel Files
You’ve already learned how to read and write Excel files with Pandas. However, there are a few more options worth considering. For one, when you use
.to_excel()
, you can specify the name of the target worksheet with the optional parameter sheet_name
:>>>
>>> df = pd.DataFrame(data=data).T
>>> df.to_excel('data.xlsx', sheet_name='COUNTRIES')
Here, you create a file
data.xlsx
with a worksheet called COUNTRIES
that stores the data. The string 'data.xlsx'
is the argument for the parameter excel_writer
that defines the name of the Excel file or its path. The optional parameters
startrow
and startcol
both default to 0
and indicate the upper left-most cell where the data should start being written:>>>
>>> df.to_excel('data-shifted.xlsx', sheet_name='COUNTRIES',
... startrow=2, startcol=4)
Here, you specify that the table should start in the third row and the fifth column. You also used zero-based indexing, so the third row is denoted by
2
and the fifth column by 4
. Now the resulting worksheet looks like this:
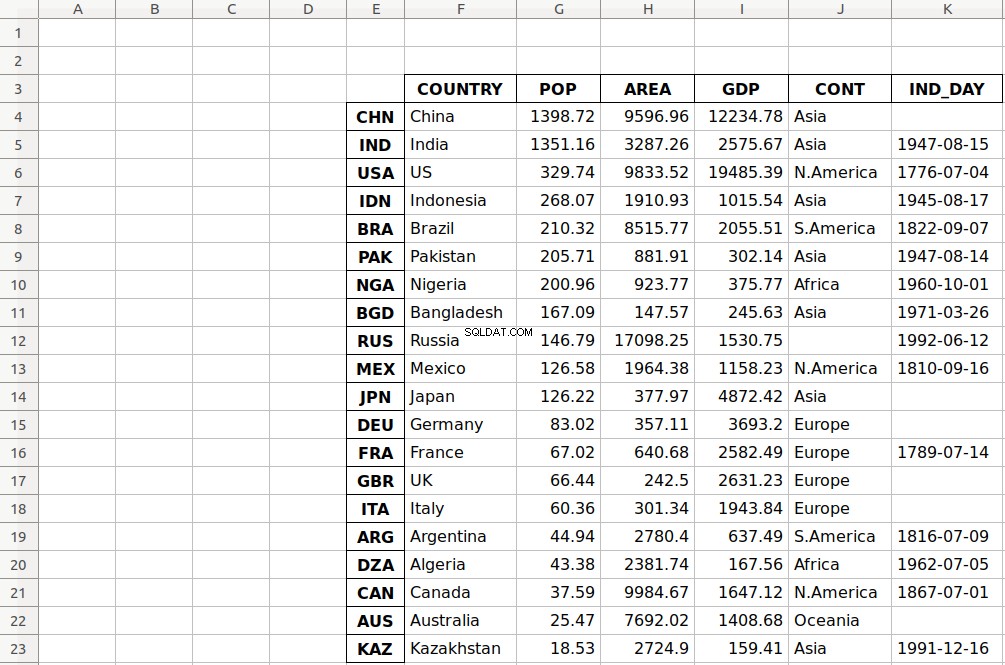
As you can see, the table starts in the third row
2
and the fifth column E
. .read_excel()
also has the optional parameter sheet_name
that specifies which worksheets to read when loading data. It can take on one of the following values:- The zero-based index of the worksheet
- The name of the worksheet
- The list of indices or names to read multiple sheets
- The value
None
to read all sheets
Here’s how you would use this parameter in your code:
>>>
>>> df = pd.read_excel('data.xlsx', sheet_name=0, index_col=0,
... parse_dates=['IND_DAY'])
>>> df = pd.read_excel('data.xlsx', sheet_name='COUNTRIES', index_col=0,
... parse_dates=['IND_DAY'])
Both statements above create the same
DataFrame
because the sheet_name
parameters have the same values. In both cases, sheet_name=0
and sheet_name='COUNTRIES'
refer to the same worksheet. The argument parse_dates=['IND_DAY']
tells Pandas to try to consider the values in this column as dates or times. There are other optional parameters you can use with
.read_excel()
and .to_excel()
to determine the Excel engine, the encoding, the way to handle missing values and infinities, the method for writing column names and row labels, and so on. SQL Files
Pandas IO tools can also read and write databases. In this next example, you’ll write your data to a database called
data.db
. To get started, you’ll need the SQLAlchemy package. To learn more about it, you can read the official ORM tutorial. You’ll also need the database driver. Python has a built-in driver for SQLite. You can install SQLAlchemy with pip:
$ pip install sqlalchemy
You can also install it with Conda:
$ conda install sqlalchemy
Once you have SQLAlchemy installed, import
create_engine()
and create a database engine:>>>
>>> from sqlalchemy import create_engine
>>> engine = create_engine('sqlite:///data.db', echo=False)
Now that you have everything set up, the next step is to create a
DataFrame
objeto. It’s convenient to specify the data types and apply .to_sql()
. >>>
>>> dtypes = {'POP': 'float64', 'AREA': 'float64', 'GDP': 'float64',
... 'IND_DAY': 'datetime64'}
>>> df = pd.DataFrame(data=data).T.astype(dtype=dtypes)
>>> df.dtypes
COUNTRY object
POP float64
AREA float64
GDP float64
CONT object
IND_DAY datetime64[ns]
dtype: object
.astype()
is a very convenient method you can use to set multiple data types at once. Once you’ve created your
DataFrame
, you can save it to the database with .to_sql()
:>>>
>>> df.to_sql('data.db', con=engine, index_label='ID')
The parameter
con
is used to specify the database connection or engine that you want to use. The optional parameter index_label
specifies how to call the database column with the row labels. You’ll often see it take on the value ID
, Id
, or id
. You should get the database
data.db
with a single table that looks like this: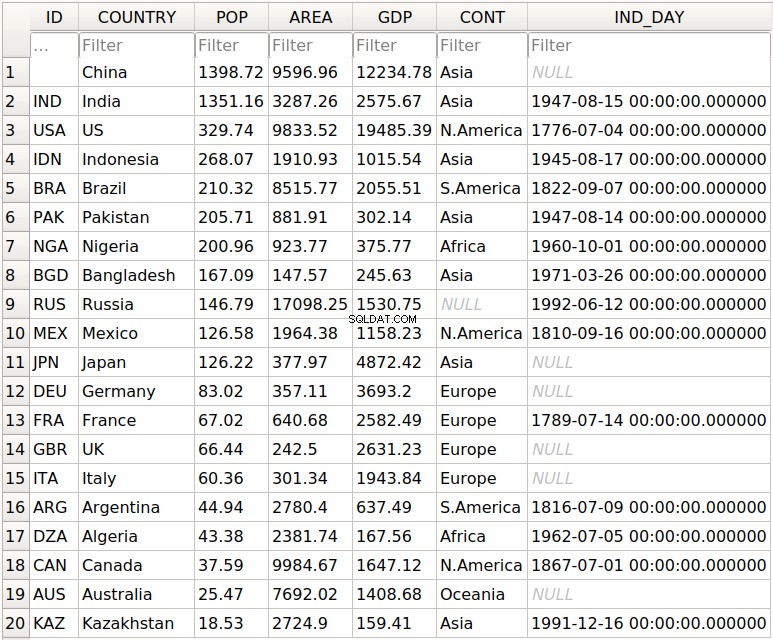
The first column contains the row labels. To omit writing them into the database, pass
index=False
to .to_sql()
. The other columns correspond to the columns of the DataFrame
. There are a few more optional parameters. For example, you can use
schema
to specify the database schema and dtype
to determine the types of the database columns. You can also use if_exists
, which says what to do if a database with the same name and path already exists:if_exists='fail'
raises a ValueError and is the default.if_exists='replace'
drops the table and inserts new values.if_exists='append'
inserts new values into the table.
You can load the data from the database with
read_sql()
:>>>
>>> df = pd.read_sql('data.db', con=engine, index_col='ID')
>>> df
COUNTRY POP AREA GDP CONT IND_DAY
ID
CHN China 1398.72 9596.96 12234.78 Asia NaT
IND India 1351.16 3287.26 2575.67 Asia 1947-08-15
USA US 329.74 9833.52 19485.39 N.America 1776-07-04
IDN Indonesia 268.07 1910.93 1015.54 Asia 1945-08-17
BRA Brazil 210.32 8515.77 2055.51 S.America 1822-09-07
PAK Pakistan 205.71 881.91 302.14 Asia 1947-08-14
NGA Nigeria 200.96 923.77 375.77 Africa 1960-10-01
BGD Bangladesh 167.09 147.57 245.63 Asia 1971-03-26
RUS Russia 146.79 17098.25 1530.75 None 1992-06-12
MEX Mexico 126.58 1964.38 1158.23 N.America 1810-09-16
JPN Japan 126.22 377.97 4872.42 Asia NaT
DEU Germany 83.02 357.11 3693.20 Europe NaT
FRA France 67.02 640.68 2582.49 Europe 1789-07-14
GBR UK 66.44 242.50 2631.23 Europe NaT
ITA Italy 60.36 301.34 1943.84 Europe NaT
ARG Argentina 44.94 2780.40 637.49 S.America 1816-07-09
DZA Algeria 43.38 2381.74 167.56 Africa 1962-07-05
CAN Canada 37.59 9984.67 1647.12 N.America 1867-07-01
AUS Australia 25.47 7692.02 1408.68 Oceania NaT
KAZ Kazakhstan 18.53 2724.90 159.41 Asia 1991-12-16
The parameter
index_col
specifies the name of the column with the row labels. Note that this inserts an extra row after the header that starts with ID
. You can fix this behavior with the following line of code:>>>
>>> df.index.name = None
>>> df
COUNTRY POP AREA GDP CONT IND_DAY
CHN China 1398.72 9596.96 12234.78 Asia NaT
IND India 1351.16 3287.26 2575.67 Asia 1947-08-15
USA US 329.74 9833.52 19485.39 N.America 1776-07-04
IDN Indonesia 268.07 1910.93 1015.54 Asia 1945-08-17
BRA Brazil 210.32 8515.77 2055.51 S.America 1822-09-07
PAK Pakistan 205.71 881.91 302.14 Asia 1947-08-14
NGA Nigeria 200.96 923.77 375.77 Africa 1960-10-01
BGD Bangladesh 167.09 147.57 245.63 Asia 1971-03-26
RUS Russia 146.79 17098.25 1530.75 None 1992-06-12
MEX Mexico 126.58 1964.38 1158.23 N.America 1810-09-16
JPN Japan 126.22 377.97 4872.42 Asia NaT
DEU Germany 83.02 357.11 3693.20 Europe NaT
FRA France 67.02 640.68 2582.49 Europe 1789-07-14
GBR UK 66.44 242.50 2631.23 Europe NaT
ITA Italy 60.36 301.34 1943.84 Europe NaT
ARG Argentina 44.94 2780.40 637.49 S.America 1816-07-09
DZA Algeria 43.38 2381.74 167.56 Africa 1962-07-05
CAN Canada 37.59 9984.67 1647.12 N.America 1867-07-01
AUS Australia 25.47 7692.02 1408.68 Oceania NaT
KAZ Kazakhstan 18.53 2724.90 159.41 Asia 1991-12-16
Now you have the same
DataFrame
object as before. Note that the continent for Russia is now
None
instead of nan
. If you want to fill the missing values with nan
, then you can use .fillna()
:>>>
>>> df.fillna(value=float('nan'), inplace=True)
.fillna()
replaces all missing values with whatever you pass to value
. Here, you passed float('nan')
, which says to fill all missing values with nan
. Also note that you didn’t have to pass
parse_dates=['IND_DAY']
to read_sql()
. That’s because your database was able to detect that the last column contains dates. However, you can pass parse_dates
if you’d like. You’ll get the same results. There are other functions that you can use to read databases, like
read_sql_table()
and read_sql_query()
. Feel free to try them out! Pickle Files
Pickling is the act of converting Python objects into byte streams. Unpickling is the inverse process. Python pickle files are the binary files that keep the data and hierarchy of Python objects. They usually have the extension
.pickle
or .pkl
. You can save your
DataFrame
in a pickle file with .to_pickle()
:>>>
>>> dtypes = {'POP': 'float64', 'AREA': 'float64', 'GDP': 'float64',
... 'IND_DAY': 'datetime64'}
>>> df = pd.DataFrame(data=data).T.astype(dtype=dtypes)
>>> df.to_pickle('data.pickle')
Like you did with databases, it can be convenient first to specify the data types. Then, you create a file
data.pickle
to contain your data. You could also pass an integer value to the optional parameter protocol
, which specifies the protocol of the pickler. You can get the data from a pickle file with
read_pickle()
:>>>
>>> df = pd.read_pickle('data.pickle')
>>> df
COUNTRY POP AREA GDP CONT IND_DAY
CHN China 1398.72 9596.96 12234.78 Asia NaT
IND India 1351.16 3287.26 2575.67 Asia 1947-08-15
USA US 329.74 9833.52 19485.39 N.America 1776-07-04
IDN Indonesia 268.07 1910.93 1015.54 Asia 1945-08-17
BRA Brazil 210.32 8515.77 2055.51 S.America 1822-09-07
PAK Pakistan 205.71 881.91 302.14 Asia 1947-08-14
NGA Nigeria 200.96 923.77 375.77 Africa 1960-10-01
BGD Bangladesh 167.09 147.57 245.63 Asia 1971-03-26
RUS Russia 146.79 17098.25 1530.75 NaN 1992-06-12
MEX Mexico 126.58 1964.38 1158.23 N.America 1810-09-16
JPN Japan 126.22 377.97 4872.42 Asia NaT
DEU Germany 83.02 357.11 3693.20 Europe NaT
FRA France 67.02 640.68 2582.49 Europe 1789-07-14
GBR UK 66.44 242.50 2631.23 Europe NaT
ITA Italy 60.36 301.34 1943.84 Europe NaT
ARG Argentina 44.94 2780.40 637.49 S.America 1816-07-09
DZA Algeria 43.38 2381.74 167.56 Africa 1962-07-05
CAN Canada 37.59 9984.67 1647.12 N.America 1867-07-01
AUS Australia 25.47 7692.02 1408.68 Oceania NaT
KAZ Kazakhstan 18.53 2724.90 159.41 Asia 1991-12-16
read_pickle()
returns the DataFrame
with the stored data. You can also check the data types:>>>
>>> df.dtypes
COUNTRY object
POP float64
AREA float64
GDP float64
CONT object
IND_DAY datetime64[ns]
dtype: object
These are the same ones that you specified before using
.to_pickle()
. As a word of caution, you should always beware of loading pickles from untrusted sources. This can be dangerous! When you unpickle an untrustworthy file, it could execute arbitrary code on your machine, gain remote access to your computer, or otherwise exploit your device in other ways.
Working With Big Data
If your files are too large for saving or processing, then there are several approaches you can take to reduce the required disk space:
- Compress your files
- Choose only the columns you want
- Omit the rows you don’t need
- Force the use of less precise data types
- Split the data into chunks
You’ll take a look at each of these techniques in turn.
Compress and Decompress Files
You can create an archive file like you would a regular one, with the addition of a suffix that corresponds to the desired compression type:
'.gz'
'.bz2'
'.zip'
'.xz'
Pandas can deduce the compression type by itself:
>>>
>>> df = pd.DataFrame(data=data).T
>>> df.to_csv('data.csv.zip')
Here, you create a compressed
.csv
file as an archive. The size of the regular .csv
file is 1048 bytes, while the compressed file only has 766 bytes. You can open this compressed file as usual with the Pandas
read_csv()
função:>>>
>>> df = pd.read_csv('data.csv.zip', index_col=0,
... parse_dates=['IND_DAY'])
>>> df
COUNTRY POP AREA GDP CONT IND_DAY
CHN China 1398.72 9596.96 12234.78 Asia NaT
IND India 1351.16 3287.26 2575.67 Asia 1947-08-15
USA US 329.74 9833.52 19485.39 N.America 1776-07-04
IDN Indonesia 268.07 1910.93 1015.54 Asia 1945-08-17
BRA Brazil 210.32 8515.77 2055.51 S.America 1822-09-07
PAK Pakistan 205.71 881.91 302.14 Asia 1947-08-14
NGA Nigeria 200.96 923.77 375.77 Africa 1960-10-01
BGD Bangladesh 167.09 147.57 245.63 Asia 1971-03-26
RUS Russia 146.79 17098.25 1530.75 NaN 1992-06-12
MEX Mexico 126.58 1964.38 1158.23 N.America 1810-09-16
JPN Japan 126.22 377.97 4872.42 Asia NaT
DEU Germany 83.02 357.11 3693.20 Europe NaT
FRA France 67.02 640.68 2582.49 Europe 1789-07-14
GBR UK 66.44 242.50 2631.23 Europe NaT
ITA Italy 60.36 301.34 1943.84 Europe NaT
ARG Argentina 44.94 2780.40 637.49 S.America 1816-07-09
DZA Algeria 43.38 2381.74 167.56 Africa 1962-07-05
CAN Canada 37.59 9984.67 1647.12 N.America 1867-07-01
AUS Australia 25.47 7692.02 1408.68 Oceania NaT
KAZ Kazakhstan 18.53 2724.90 159.41 Asia 1991-12-16
read_csv()
decompresses the file before reading it into a DataFrame
. You can specify the type of compression with the optional parameter
compression
, which can take on any of the following values:'infer'
'gzip'
'bz2'
'zip'
'xz'
None
The default value
compression='infer'
indicates that Pandas should deduce the compression type from the file extension. Here’s how you would compress a pickle file:
>>>
>>> df = pd.DataFrame(data=data).T
>>> df.to_pickle('data.pickle.compress', compression='gzip')
You should get the file
data.pickle.compress
that you can later decompress and read:>>>
>>> df = pd.read_pickle('data.pickle.compress', compression='gzip')
df
again corresponds to the DataFrame
with the same data as before. You can give the other compression methods a try, as well. If you’re using pickle files, then keep in mind that the
.zip
format supports reading only. Choose Columns
The Pandas
read_csv()
and read_excel()
functions have the optional parameter usecols
that you can use to specify the columns you want to load from the file. You can pass the list of column names as the corresponding argument:>>>
>>> df = pd.read_csv('data.csv', usecols=['COUNTRY', 'AREA'])
>>> df
COUNTRY AREA
0 China 9596.96
1 India 3287.26
2 US 9833.52
3 Indonesia 1910.93
4 Brazil 8515.77
5 Pakistan 881.91
6 Nigeria 923.77
7 Bangladesh 147.57
8 Russia 17098.25
9 Mexico 1964.38
10 Japan 377.97
11 Germany 357.11
12 France 640.68
13 UK 242.50
14 Italy 301.34
15 Argentina 2780.40
16 Algeria 2381.74
17 Canada 9984.67
18 Australia 7692.02
19 Kazakhstan 2724.90
Now you have a
DataFrame
that contains less data than before. Here, there are only the names of the countries and their areas. Instead of the column names, you can also pass their indices:
>>>
>>> df = pd.read_csv('data.csv',index_col=0, usecols=[0, 1, 3])
>>> df
COUNTRY AREA
CHN China 9596.96
IND India 3287.26
USA US 9833.52
IDN Indonesia 1910.93
BRA Brazil 8515.77
PAK Pakistan 881.91
NGA Nigeria 923.77
BGD Bangladesh 147.57
RUS Russia 17098.25
MEX Mexico 1964.38
JPN Japan 377.97
DEU Germany 357.11
FRA France 640.68
GBR UK 242.50
ITA Italy 301.34
ARG Argentina 2780.40
DZA Algeria 2381.74
CAN Canada 9984.67
AUS Australia 7692.02
KAZ Kazakhstan 2724.90
Expand the code block below to compare these results with the file
'data.csv'
:,COUNTRY,POP,AREA,GDP,CONT,IND_DAY
CHN,China,1398.72,9596.96,12234.78,Asia,
IND,India,1351.16,3287.26,2575.67,Asia,1947-08-15
USA,US,329.74,9833.52,19485.39,N.America,1776-07-04
IDN,Indonesia,268.07,1910.93,1015.54,Asia,1945-08-17
BRA,Brazil,210.32,8515.77,2055.51,S.America,1822-09-07
PAK,Pakistan,205.71,881.91,302.14,Asia,1947-08-14
NGA,Nigeria,200.96,923.77,375.77,Africa,1960-10-01
BGD,Bangladesh,167.09,147.57,245.63,Asia,1971-03-26
RUS,Russia,146.79,17098.25,1530.75,,1992-06-12
MEX,Mexico,126.58,1964.38,1158.23,N.America,1810-09-16
JPN,Japan,126.22,377.97,4872.42,Asia,
DEU,Germany,83.02,357.11,3693.2,Europe,
FRA,France,67.02,640.68,2582.49,Europe,1789-07-14
GBR,UK,66.44,242.5,2631.23,Europe,
ITA,Italy,60.36,301.34,1943.84,Europe,
ARG,Argentina,44.94,2780.4,637.49,S.America,1816-07-09
DZA,Algeria,43.38,2381.74,167.56,Africa,1962-07-05
CAN,Canada,37.59,9984.67,1647.12,N.America,1867-07-01
AUS,Australia,25.47,7692.02,1408.68,Oceania,
KAZ,Kazakhstan,18.53,2724.9,159.41,Asia,1991-12-16
You can see the following columns:
- The column at index
0
contains the row labels. - The column at index
1
contains the country names. - The column at index
3
contains the areas.
Simlarly,
read_sql()
has the optional parameter columns
that takes a list of column names to read:>>>
>>> df = pd.read_sql('data.db', con=engine, index_col='ID',
... columns=['COUNTRY', 'AREA'])
>>> df.index.name = None
>>> df
COUNTRY AREA
CHN China 9596.96
IND India 3287.26
USA US 9833.52
IDN Indonesia 1910.93
BRA Brazil 8515.77
PAK Pakistan 881.91
NGA Nigeria 923.77
BGD Bangladesh 147.57
RUS Russia 17098.25
MEX Mexico 1964.38
JPN Japan 377.97
DEU Germany 357.11
FRA France 640.68
GBR UK 242.50
ITA Italy 301.34
ARG Argentina 2780.40
DZA Algeria 2381.74
CAN Canada 9984.67
AUS Australia 7692.02
KAZ Kazakhstan 2724.90
Again, the
DataFrame
only contains the columns with the names of the countries and areas. If columns
is None
or omitted, then all of the columns will be read, as you saw before. The default behavior is columns=None
. Omit Rows
When you test an algorithm for data processing or machine learning, you often don’t need the entire dataset. It’s convenient to load only a subset of the data to speed up the process. The Pandas
read_csv()
and read_excel()
functions have some optional parameters that allow you to select which rows you want to load:skiprows
: either the number of rows to skip at the beginning of the file if it’s an integer, or the zero-based indices of the rows to skip if it’s a list-like objectskipfooter
: the number of rows to skip at the end of the filenrows
: the number of rows to read
Here’s how you would skip rows with odd zero-based indices, keeping the even ones:
>>>
>>> df = pd.read_csv('data.csv', index_col=0, skiprows=range(1, 20, 2))
>>> df
COUNTRY POP AREA GDP CONT IND_DAY
IND India 1351.16 3287.26 2575.67 Asia 1947-08-15
IDN Indonesia 268.07 1910.93 1015.54 Asia 1945-08-17
PAK Pakistan 205.71 881.91 302.14 Asia 1947-08-14
BGD Bangladesh 167.09 147.57 245.63 Asia 1971-03-26
MEX Mexico 126.58 1964.38 1158.23 N.America 1810-09-16
DEU Germany 83.02 357.11 3693.20 Europe NaN
GBR UK 66.44 242.50 2631.23 Europe NaN
ARG Argentina 44.94 2780.40 637.49 S.America 1816-07-09
CAN Canada 37.59 9984.67 1647.12 N.America 1867-07-01
KAZ Kazakhstan 18.53 2724.90 159.41 Asia 1991-12-16
In this example,
skiprows
is range(1, 20, 2)
and corresponds to the values 1
, 3
, …, 19
. The instances of the Python built-in class range
behave like sequences. The first row of the file data.csv
is the header row. It has the index 0
, so Pandas loads it in. The second row with index 1
corresponds to the label CHN
, and Pandas skips it. The third row with the index 2
and label IND
is loaded, and so on. If you want to choose rows randomly, then
skiprows
can be a list or NumPy array with pseudo-random numbers, obtained either with pure Python or with NumPy. Force Less Precise Data Types
If you’re okay with less precise data types, then you can potentially save a significant amount of memory! First, get the data types with
.dtypes
novamente:>>>
>>> df = pd.read_csv('data.csv', index_col=0, parse_dates=['IND_DAY'])
>>> df.dtypes
COUNTRY object
POP float64
AREA float64
GDP float64
CONT object
IND_DAY datetime64[ns]
dtype: object
The columns with the floating-point numbers are 64-bit floats. Each number of this type
float64
consumes 64 bits or 8 bytes. Each column has 20 numbers and requires 160 bytes. You can verify this with .memory_usage()
:>>>
>>> df.memory_usage()
Index 160
COUNTRY 160
POP 160
AREA 160
GDP 160
CONT 160
IND_DAY 160
dtype: int64
.memory_usage()
returns an instance of Series
with the memory usage of each column in bytes. You can conveniently combine it with .loc[]
and .sum()
to get the memory for a group of columns:>>>
>>> df.loc[:, ['POP', 'AREA', 'GDP']].memory_usage(index=False).sum()
480
This example shows how you can combine the numeric columns
'POP'
, 'AREA'
, and 'GDP'
to get their total memory requirement. The argument index=False
excludes data for row labels from the resulting Series
objeto. For these three columns, you’ll need 480 bytes. You can also extract the data values in the form of a NumPy array with
.to_numpy()
or .values
. Then, use the .nbytes
attribute to get the total bytes consumed by the items of the array:>>>
>>> df.loc[:, ['POP', 'AREA', 'GDP']].to_numpy().nbytes
480
The result is the same 480 bytes. So, how do you save memory?
In this case, you can specify that your numeric columns
'POP'
, 'AREA'
, and 'GDP'
should have the type float32
. Use the optional parameter dtype
to do this:>>>
>>> dtypes = {'POP': 'float32', 'AREA': 'float32', 'GDP': 'float32'}
>>> df = pd.read_csv('data.csv', index_col=0, dtype=dtypes,
... parse_dates=['IND_DAY'])
The dictionary
dtypes
specifies the desired data types for each column. It’s passed to the Pandas read_csv()
function as the argument that corresponds to the parameter dtype
. Now you can verify that each numeric column needs 80 bytes, or 4 bytes per item:
>>>
>>> df.dtypes
COUNTRY object
POP float32
AREA float32
GDP float32
CONT object
IND_DAY datetime64[ns]
dtype: object
>>> df.memory_usage()
Index 160
COUNTRY 160
POP 80
AREA 80
GDP 80
CONT 160
IND_DAY 160
dtype: int64
>>> df.loc[:, ['POP', 'AREA', 'GDP']].memory_usage(index=False).sum()
240
>>> df.loc[:, ['POP', 'AREA', 'GDP']].to_numpy().nbytes
240
Each value is a floating-point number of 32 bits or 4 bytes. The three numeric columns contain 20 items each. In total, you’ll need 240 bytes of memory when you work with the type
float32
. This is half the size of the 480 bytes you’d need to work with float64
. In addition to saving memory, you can significantly reduce the time required to process data by using
float32
instead of float64
in some cases. Use Chunks to Iterate Through Files
Another way to deal with very large datasets is to split the data into smaller chunks and process one chunk at a time. If you use
read_csv()
, read_json()
or read_sql()
, then you can specify the optional parameter chunksize
:>>>
>>> data_chunk = pd.read_csv('data.csv', index_col=0, chunksize=8)
>>> type(data_chunk)
<class 'pandas.io.parsers.TextFileReader'>
>>> hasattr(data_chunk, '__iter__')
True
>>> hasattr(data_chunk, '__next__')
True
chunksize
defaults to None
and can take on an integer value that indicates the number of items in a single chunk. When chunksize
is an integer, read_csv()
returns an iterable that you can use in a for
loop to get and process only a fragment of the dataset in each iteration:>>>
>>> for df_chunk in pd.read_csv('data.csv', index_col=0, chunksize=8):
... print(df_chunk, end='\n\n')
... print('memory:', df_chunk.memory_usage().sum(), 'bytes',
... end='\n\n\n')
...
COUNTRY POP AREA GDP CONT IND_DAY
CHN China 1398.72 9596.96 12234.78 Asia NaN
IND India 1351.16 3287.26 2575.67 Asia 1947-08-15
USA US 329.74 9833.52 19485.39 N.America 1776-07-04
IDN Indonesia 268.07 1910.93 1015.54 Asia 1945-08-17
BRA Brazil 210.32 8515.77 2055.51 S.America 1822-09-07
PAK Pakistan 205.71 881.91 302.14 Asia 1947-08-14
NGA Nigeria 200.96 923.77 375.77 Africa 1960-10-01
BGD Bangladesh 167.09 147.57 245.63 Asia 1971-03-26
memory: 448 bytes
COUNTRY POP AREA GDP CONT IND_DAY
RUS Russia 146.79 17098.25 1530.75 NaN 1992-06-12
MEX Mexico 126.58 1964.38 1158.23 N.America 1810-09-16
JPN Japan 126.22 377.97 4872.42 Asia NaN
DEU Germany 83.02 357.11 3693.20 Europe NaN
FRA France 67.02 640.68 2582.49 Europe 1789-07-14
GBR UK 66.44 242.50 2631.23 Europe NaN
ITA Italy 60.36 301.34 1943.84 Europe NaN
ARG Argentina 44.94 2780.40 637.49 S.America 1816-07-09
memory: 448 bytes
COUNTRY POP AREA GDP CONT IND_DAY
DZA Algeria 43.38 2381.74 167.56 Africa 1962-07-05
CAN Canada 37.59 9984.67 1647.12 N.America 1867-07-01
AUS Australia 25.47 7692.02 1408.68 Oceania NaN
KAZ Kazakhstan 18.53 2724.90 159.41 Asia 1991-12-16
memory: 224 bytes
In this example, the
chunksize
is 8
. The first iteration of the for
loop returns a DataFrame
with the first eight rows of the dataset only. The second iteration returns another DataFrame
with the next eight rows. The third and last iteration returns the remaining four rows. Observação: You can also pass
iterator=True
to force the Pandas read_csv()
function to return an iterator object instead of a DataFrame
objeto. In each iteration, you get and process the
DataFrame
with the number of rows equal to chunksize
. It’s possible to have fewer rows than the value of chunksize
in the last iteration. You can use this functionality to control the amount of memory required to process data and keep that amount reasonably small. Conclusão
You now know how to save the data and labels from Pandas
DataFrame
objects to different kinds of files. You also know how to load your data from files and create DataFrame
objetos. You’ve used the Pandas
read_csv()
and .to_csv()
methods to read and write CSV files. You also used similar methods to read and write Excel, JSON, HTML, SQL, and pickle files. These functions are very convenient and widely used. They allow you to save or load your data in a single function or method call. You’ve also learned how to save time, memory, and disk space when working with large data files:
- Compress or decompress files
- Choose the rows and columns you want to load
- Use less precise data types
- Split data into chunks and process them one by one
You’ve mastered a significant step in the machine learning and data science process! If you have any questions or comments, then please put them in the comments section below.